(CDRGI)-Cancer detection through relevant genes identification
Document Type
Article
Source of Publication
Neural Computing and Applications
Publication Date
2-5-2021
Abstract
Cancer is a genetic disease that is categorized among the most lethal and belligerent diseases. An early staging of the disease can reduce the high mortality rate associated with cancer. The advancement in high throughput sequencing technology and the implementation of several Machine Learning algorithms have led to significant progress in Oncogenomics over the past few decades. Oncogenomics uses RNA sequencing and gene expression profiling for the identification of cancer-related genes. The high dimensionality of RNA sequencing data makes it a complex and large-scale optimization problem. CDRGI presents a Discrete Filtering technique based on a Binary Artificial Bee Colony coupling Support Vector Machine and a two-stage cascading classifier to identify relevant genes and detect cancer using RNA seq data. The proposed approach has been tested for seven different cancers, including Breast Cancer, Stomach Cancer (STAD), Colon Cancer (COAD), Liver Cancer, Lung Cancer (LUSC), Kidney Cancer (KIRC), and Skin Cancer. The results revealed that the CDRGI performs better for feature reduction while achieving better classification accuracy for STAD, COAD, LUSC and KIRC cancer types.
DOI Link
ISSN
Publisher
Springer Nature
Disciplines
Medicine and Health Sciences
Keywords
Support vector machine, Cascading classifier, Discrete filtering, Artificial bee colony, Gene expression, CatBoost classifier, Convolutional neural network
Scopus ID
Recommended Citation
Al-Obeidat, Feras; Rocha, Álvaro; Akram, Maryam; Razzaq, Saad; and Maqbool, Fahad, "(CDRGI)-Cancer detection through relevant genes identification" (2021). All Works. 17.
https://zuscholars.zu.ac.ae/works/17
Indexed in Scopus
yes
Open Access
no
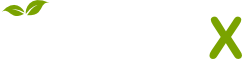
- Citations
- Citation Indexes: 3
- Usage
- Abstract Views: 16
- Captures
- Readers: 28
- Mentions
- News Mentions: 1