ORCID Identifiers
Document Type
Article
Source of Publication
Algorithms
Publication Date
5-1-2019
Abstract
© 2019 by the authors. Domain adaptation is a sub-field of transfer learning that aims at bridging the dissimilarity gap between different domains by transferring and re-using the knowledge obtained in the source domain to the target domain. Many methods have been proposed to resolve this problem, using techniques such as generative adversarial networks (GAN), but the complexity of such methods makes it hard to use them in different problems, as fine-tuning such networks is usually a time-consuming task. In this paper, we propose a method for unsupervised domain adaptation that is both simple and effective. Our model (referred to as TripNet) harnesses the idea of a discriminator and Linear Discriminant Analysis (LDA) to push the encoder to generate domain-invariant features that are category-informative. At the same time, pseudo-labelling is used for the target data to train the classifier and to bring the same classes from both domains together. We evaluate TripNet against several existing, state-of-the-art methods on three image classification tasks: Digit classification (MNIST, SVHN, and USPC datasets), object recognition (Office31 dataset), and traffic sign recognition (GTSRB and Synthetic Signs datasets). Our experimental results demonstrate that (i) TripNet beats almost all existing methods (having a similar simple model like it) on all of these tasks; and (ii) for models that are significantly more complex (or hard to train) than TripNet, it even beats their performance in some cases. Hence, the results confirm the effectiveness of using TripNet for unsupervised domain adaptation in image classification.
DOI Link
ISSN
Publisher
MDPI AG
Volume
12
Issue
5
Disciplines
Computer Sciences
Keywords
Adversarial loss, Computer vision, Deep learning, Domain adaptation, Linear discriminant analysis, Representation learning, Transfer learning
Scopus ID
Creative Commons License
This work is licensed under a Creative Commons Attribution 4.0 International License.
Recommended Citation
Bekkouch, Imad Eddine Ibrahim; Youssry, Youssef; Gafarov, Rustam; Khan, Adil; and Khattak, Asad Masood, "Triplet loss network for unsupervised domain adaptation" (2019). All Works. 3783.
https://zuscholars.zu.ac.ae/works/3783
Indexed in Scopus
yes
Open Access
yes
Open Access Type
Gold: This publication is openly available in an open access journal/series
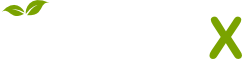
- Citations
- Citation Indexes: 13
- Patent Family Citations: 1
- Usage
- Downloads: 56
- Abstract Views: 1
- Captures
- Readers: 35