On the Feasibility of Split Learning, Transfer Learning and Federated Learning for Preserving Security in ITS Systems
Document Type
Article
Source of Publication
IEEE Transactions on Intelligent Transportation Systems
Publication Date
1-1-2022
Abstract
Due to the absence of distinct boundaries, wireless networks are vulnerable to a variety of intrusions. As the number of intruders has increased, the risks on critical infrastructures monitored by networked systems have also increased. Protecting shared information using effective and robust Intrusion Detection Systems (IDSs) remains a critical issue, especially with the growing implementation of vehicular networks. Building an IDS that detects threats efficiently with maximum accuracy and detection is a challenging undertaking. Machine Learning (ML) mechanisms have been successfully adopted in IDSs to detect a variety of network intruders. Split learning is considered one of the main developments in creating efficient ML approaches. In utilizing the Split Learning approach, an IDS is successful in performing at higher accuracy, and detection rate as well as a higher classification performance (Precision, Recall). In this work, a Split Learning-based IDS (SplitLearn) for Intelligent Transportation System (ITS) infrastructures has been proposed to address the potential security concerns. The proposed model has been evaluated and compared against other models (i.e., Federated Learning (FedLearn) and Transfer Learning (TransLearn)-based solutions). With the highest accuracy and detection rates, the proposed model (SplitLearn) outperforms FedLearn and TransLearn by 2 to 5 % respectively. We also see a decrease in power consumption when utilizing SplitLearn versus FedLearn.
DOI Link
ISSN
Publisher
Institute of Electrical and Electronics Engineers (IEEE)
Volume
PP
Issue
99
First Page
1
Last Page
9
Disciplines
Computer Sciences
Keywords
Transfer learning, Security, Collaborative work, Medical services, Task analysis, Monitoring, Industrial Internet of Things
Recommended Citation
Otoum, Safa; Guizani, Nadra; and Mouftah, Hussein, "On the Feasibility of Split Learning, Transfer Learning and Federated Learning for Preserving Security in ITS Systems" (2022). All Works. 5002.
https://zuscholars.zu.ac.ae/works/5002
Indexed in Scopus
no
Open Access
no
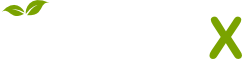
- Citations
- Citation Indexes: 24
- Usage
- Abstract Views: 8
- Captures
- Readers: 51
- Mentions
- News Mentions: 1