Document Type
Article
Source of Publication
Applied System Innovation
Publication Date
9-29-2023
Abstract
The utilization of data mining techniques for the prompt prediction of academic success has gained significant importance in the current era. There is an increasing interest in utilizing these methodologies to forecast the academic performance of students, thereby facilitating educators to intervene and furnish suitable assistance when required. The purpose of this study was to determine the optimal methods for feature engineering and selection in the context of regression and classification tasks. This study compared the Boruta algorithm and Lasso regression for regression, and Recursive Feature Elimination (RFE) and Random Forest Importance (RFI) for classification. According to the findings, Gradient Boost for the regression part of this study had the least Mean Absolute Error (MAE) and Root-Mean-Square Error (RMSE) of 12.93 and 18.28, respectively, in the case of the Boruta selection method. In contrast, RFI was found to be the superior classification method, yielding an accuracy rate of 78% in the classification part. This research emphasized the significance of employing appropriate feature engineering and selection methodologies to enhance the efficacy of machine learning algorithms. Using a diverse set of machine learning techniques, this study analyzed the OULA dataset, focusing on both feature engineering and selection. Our approach was to systematically compare the performance of different models, leading to insights about the most effective strategies for predicting student success.
DOI Link
ISSN
Publisher
MDPI AG
Volume
6
Issue
5
First Page
86
Last Page
86
Disciplines
Computer Sciences
Keywords
data mining, feature selection methods, Boruta algorithm, lasso regression, recursive feature elimination (RFE), random forest importance (RFI)
Creative Commons License
This work is licensed under a Creative Commons Attribution 4.0 International License.
Recommended Citation
Syed Mustapha, S. M. F. D., "Predictive Analysis of Students’ Learning Performance Using Data Mining Techniques: A Comparative Study of Feature Selection Methods" (2023). All Works. 6163.
https://zuscholars.zu.ac.ae/works/6163
Indexed in Scopus
no
Open Access
yes
Open Access Type
Gold: This publication is openly available in an open access journal/series
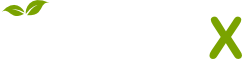
- Citations
- Citation Indexes: 21
- Usage
- Downloads: 109
- Abstract Views: 17
- Captures
- Readers: 88
- Mentions
- Blog Mentions: 1