A deep learning-assisted visual attention mechanism for anomaly detection in videos
Document Type
Article
Source of Publication
Multimedia Tools and Applications
Publication Date
1-1-2023
Abstract
Ensuring public safety in urban areas is a crucial element in maintaining a good quality of life. The successful deployment of video surveillance systems depends heavily on the acquisition and processing of large volumes of urban data to derive meaningful insights. Manual monitoring and analysis of anomalous activities in the surveillance footage is both a time-consuming and error-prone process that is not scalable for urban environments with high levels of foot and vehicular traffic. Moreover, traditional surveillance systems are limited by their inability to process real-time data at scale, which can result in missed or delayed detection of potential security threats. This paper tackles this problem by proposing an automatic anomaly detection method via an attention mechanism. The attention area is identified using the background subtraction (BG) algorithm which identifies motion regions in the video frames. This information is then passed through a 3D convolutional neural network (3D CNN) to classify the normal and anomalous events. To evaluate the proposed method, experiments and analysis were conducted using the publicly available UCF crime dataset, demonstrating its effectiveness with an accuracy of 96.89% compared to the state-of-the-art methods. In case an anomaly is detected, an alert is sent to the nearest authorities to take immediate action to prevent further harm or damage.
DOI Link
ISSN
Publisher
Springer Science and Business Media LLC
Disciplines
Computer Sciences
Keywords
Attention mechanism, Background subtraction, Deep learning, Transfer learning, Violence detection
Scopus ID
Recommended Citation
Shoaib, Muhammad; Shah, Babar; Hussain, Tariq; Yang, Bailin; Ullah, Asad; Khan, Jahangir; and Ali, Farman, "A deep learning-assisted visual attention mechanism for anomaly detection in videos" (2023). All Works. 6240.
https://zuscholars.zu.ac.ae/works/6240
Indexed in Scopus
yes
Open Access
no
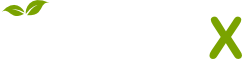
- Citations
- Citation Indexes: 2
- Usage
- Abstract Views: 6
- Captures
- Readers: 11
- Mentions
- News Mentions: 1