Enhancing arrhythmia prediction through an adaptive deep reinforcement learning framework for ECG signal analysis
Document Type
Article
Source of Publication
Biomedical Signal Processing and Control
Publication Date
3-1-2025
Abstract
Heart diseases, particularly arrhythmia, remain a leading global cause of mortality. Electrocardiography (ECG) serves as a vital tool for monitoring and mitigating arrhythmia risks. Continuous proactive monitoring entails processing ECG time series data, offering critical insights to prevent severe outcomes like heart attacks and strokes. While deep learning models are widely employed for large-scale ECG data analysis, their effectiveness requires intricate hyperparameter tuning and architecture adjustments. This study presents an innovative framework incorporating a reinforcement learning-based approach to automatically fine-tune hyperparameters for a Convolutional Neural Network (CNN) model, addressing resource consumption and complexity issues. Our proposed model optimizes hyperparameters and network configurations by maximizing a reward function, overcoming challenges associated with resource-intensive and complex deep learning models. To assess its efficacy, we compare our automated fine-tuning model against both a non-optimized baseline model and a manually fine-tuned model. The findings demonstrate that our model outperforms the alternatives, achieving a higher accuracy of 97.4 %, reduced execution time (0.33 min), and lower mean square error (0.0081). Moreover, a comprehensive evaluation of resource consumption illustrates the lightweight nature of our proposed solution, minimizing overhead and complexity. Our model consistently converges to an optimal configuration, establishing its efficiency in predicting arrhythmia. In conclusion, our reinforcement learning-based hyperparameter tuning approach presents a lightweight and efficient solution for optimizing CNN models in ECG analysis. The results underscore its superiority over non-optimized and manually fine-tuned models, positioning it as a promising method for enhancing arrhythmia prediction accuracy while minimizing resource consumption and complexity.
DOI Link
ISSN
Volume
101
Disciplines
Medicine and Health Sciences
Keywords
Agent, Arrhythmia, CNN, DEEP learning, ECG, Heart diseases, Reinforcement learning
Scopus ID
Recommended Citation
Serhani, Mohamed Adel; Ismail, Heba; El-Kassabi, Hadeel T.; and Breiki, Hamda Al, "Enhancing arrhythmia prediction through an adaptive deep reinforcement learning framework for ECG signal analysis" (2025). All Works. 6928.
https://zuscholars.zu.ac.ae/works/6928
Indexed in Scopus
yes
Open Access
no
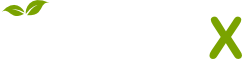
- Citations
- Citation Indexes: 1
- Usage
- Abstract Views: 4
- Captures
- Readers: 15
- Mentions
- News Mentions: 1