Document Type
Article
Source of Publication
Scientific reports
Publication Date
12-29-2022
Abstract
Smart grids and smart homes are getting people's attention in the modern era of smart cities. The advancements of smart technologies and smart grids have created challenges related to energy efficiency and production according to the future demand of clients. Machine learning, specifically neural network-based methods, remained successful in energy consumption prediction, but still, there are gaps due to uncertainty in the data and limitations of the algorithms. Research published in the literature has used small datasets and profiles of primarily single users; therefore, models have difficulties when applied to large datasets with profiles of different customers. Thus, a smart grid environment requires a model that handles consumption data from thousands of customers. The proposed model enhances the newly introduced method of Neural Basis Expansion Analysis for interpretable Time Series (N-BEATS) with a big dataset of energy consumption of 169 customers. Further, to validate the results of the proposed model, a performance comparison has been carried out with the Long Short Term Memory (LSTM), Blocked LSTM, Gated Recurrent Units (GRU), Blocked GRU and Temporal Convolutional Network (TCN). The proposed interpretable model improves the prediction accuracy on the big dataset containing energy consumption profiles of multiple customers. Incorporating covariates into the model improved accuracy by learning past and future energy consumption patterns. Based on a large dataset, the proposed model performed better for daily, weekly, and monthly energy consumption predictions. The forecasting accuracy of the N-BEATS interpretable model for 1-day-ahead energy consumption with "day as covariates" remained better than the 1, 2, 3, and 4-week scenarios.
DOI Link
ISSN
Publisher
Springer Science and Business Media LLC
Volume
12
Issue
1
First Page
22562
Disciplines
Computer Sciences
Scopus ID
Creative Commons License
This work is licensed under a Creative Commons Attribution 4.0 International License.
Recommended Citation
Shaikh, Abdul Khalique; Nazir, Amril; Khan, Imran; and Shah, Abdul Salam, "Short term energy consumption forecasting using neural basis expansion analysis for interpretable time series" (2022). All Works. 5536.
https://zuscholars.zu.ac.ae/works/5536
Indexed in Scopus
yes
Open Access
yes
Open Access Type
Gold: This publication is openly available in an open access journal/series
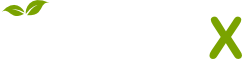
- Citations
- Citation Indexes: 27
- Patent Family Citations: 1
- Usage
- Downloads: 48
- Abstract Views: 3
- Captures
- Readers: 75